In the dynamic world of digital marketing, you are always striving to allocate your resources in such a way that maximises the impact of your media channels. Media Mix Modelling is a powerful tool that allows you to estimate the contributions of various media channels on KPIs. However, the effectiveness of Media Mix Modelling relies on accurate calibration. This is where incrementality testing comes into play, offering a data-driven approach to fine-tune Media Mix Modelling strategies.
- What is Media Mix Modelling?
- What is incrementality testing?
- Common challenges faced in Media Mix Modelling
- Capturing the effects of upper funnel advertising
- Adstock effects
- How incrementality testing can overcome these challenges
- Upper-funnel advertising solution
- Adstock effects solution
- Why calibrating your Media Mix Model is crucial
What is Media Mix Modelling?
Media Mix Modelling is a powerful statistical tool frequently used in media effectiveness. Media Mix Modelling helps to determine how different media channels and other external factors contribute towards achieving desired marketing goals such as revenue or conversions. The Media Mix Model contribution estimates can then be used to provide an optimal budget distribution. It works by analysing historical data on various media channels and their impact on KPIs. It utilises collected data on media spend, KPIs such as conversions or revenue and other context variables such as events, average product price or seasonality.
What is incrementality testing?
Incrementality testing on the other hand is a statistical method used to measure and estimate the direct impact, cumulative impact, and short/long-term effects of a campaign or particular media channel. Incrementality testing aims to establish a causal relationship by comparing a “treatment” with a “control” group. The treatment group refers to a group exposed to a marketing experiment and the control group refers to a group that hasn’t been exposed. Incrementality testing provides ways to quantify direct media impact using data, and the separation of confounding variables.
At first glance these two methods may seem independent, however, combining Media Mix Modelling with incrementality testing is key to generating a trustworthy media mix.
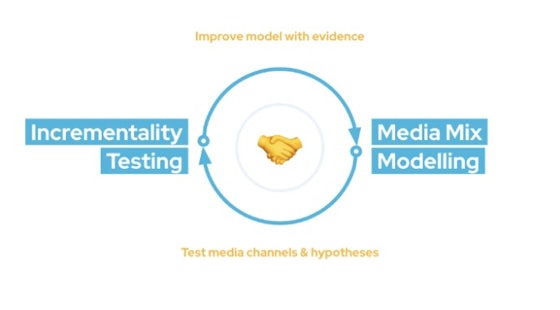
Common challenges faced in Media Mix Modelling
Capturing the effects of upper funnel advertising
In the context of Bayesian Media Mix Modelling, media contributions are modelled as a random variable characterised by a combination of prior knowledge and the data, as opposed to an unknown fixed real value.
In Bayesian Media Mix Modelling, choosing priors can be difficult depending on how much prior knowledge is available. If very little prior knowledge is available then one approach is to let the data do the talking, through the use of non-informative priors. Allowing the data to do the talking may sound like the rational thing to do at first glance, however, the Media Mix Model will distribute media contribution based on the data the model has access to and what associations it can detect within.
A common issue in Media Mix Modelling is that sometimes the spend on upper funnel advertising might not appear to be associated with revenue (often the dependent variable). However, this doesn’t always mean upper-funnel advertising is ineffective. One attempt to counter this is to increase prior contributions for that particular media channel, but doing this without any testing involves a lot of guesswork – something you wish to avoid. Additionally, having too much prior confidence can lead to inaccurate and potentially biased results. Therefore, based on this, you require a sensible method to inform oyur model.
Adstock effects
Additionally, trying to elicit prior adstock effects on a particular media channel can be challenging. Besides guesswork, one attempt is to tackle this through the use of Multi-touch Attribution Models. Multi-touch Attribution Models track the customer journey and could be used to provide approximations to the Adstock effects of media channels, provided it can track the time between touch points.
However, this is quite a contradictory approach as some of the main reasons Media Mix Modelling is becoming so popular in the world of digital marketing are the decaying lifetime of the third-party cookie, the technological challenges posed by devices staying compliant with privacy laws, and changing consumer preferences to switch between devices more and more often. As the effectiveness of the Multi-touch Attribution Model decays, the more fragmented the touch points and the customer journeys are, and hence the less confidence you have in your results. Again, based on this, you require a sensible way to inform your model.
Luckily, you have the power of incrementality testing at your disposal.
How incrementality testing can overcome these challenges
Upper-funnel advertising solution
One efficient way to capture and assess the direct effectiveness of upper-funnel advertising (whilst controlling for confounding variables) is through incrementality testing.
For example, if you decided to hold out on upper funnel advertising in one location, call this the “treatment location”, you would then use the other “control” locations to estimate what would’ve happened to the revenue generated in the “treatment” location if you didn’t hold out on upper-funnel advertising.
These estimations will define the “synthetic control” group. You then compare what actually happened to revenue, as a result of holding out on upper-funnel advertising, with what was predicted to happen. If the test indicates very little or no significant impact, then a Media Mix Model suggesting this particular channel is less effective than expected may be justified.
Alternatively, if the test indicates upper funnel advertising does have an impact on revenue, then you may increase prior contribution in such a way that aligns with the results of this test. This process is referred to as model calibration.
Adstock effects solution
Incrementality testing provides a way to measure the Adstock effects of a particular media channel.
For example, if you decide to deactivate advertising in one location for a particular media channel, call this the “treatment location”, you would then use the other “control” locations to estimate what would’ve happened to the revenue generated in the “treatment” location if you didn’t deactivate advertising.
You then compare what actually happened to revenue, as a result of deactivating advertising, with what was predicted to happen. If the test indicates that deactivating advertising on this particular media channel has significantly impacted revenue, then the time it takes to see the significant impact provides a good prior Adstock estimate to inform the model.
Why calibrating your Media Mix Model is crucial
- Incrementality testing helps to establish causal relationships, whereas a Media Mix Model alone will allocate contributions based on the correlations it can detect. Incrementality testing enables Media Mix Models to capture casual relationships more efficiently.
- Incrementality testing enables us to validate any results generated by a Media Mix Model. For example, if a previous Media Mix Model predicts that increasing spend on a particular channel will generate a specific amount of additional revenue, performing incrementality testing on this channel will allow us to check this claim.
- Incrementality testing results can be used to fine-tune the parameters of a Media Mix Model. For instance, it can help refine the contribution coefficients assigned to different marketing channels, ensuring that the model accurately captures the true impact of each channel.
- By understanding the incremental impact of each marketing channel, you can make more informed decisions about how to allocate marketing resources. This can lead to more efficient spending and increased overall business performance.
- Incrementality testing allows for the control of confounding variables that may affect the Media Mix Model. By isolating the impact of a specific media channel, you can better control for external factors that might influence outcomes.
- The world of digital marketing is dynamic, and consumer behaviour is ever-changing. Incrementality testing aids in the continuous improvement and validation of previous Media Mix Models.
The combination of both Media Mix Modelling and incrementality testing allows you to navigate the world of media effectiveness and strengthens the validity of data-driven insights. By leveraging the causal insights provided by incrementality testing, you can fine-tune your Media Mix Models, ensuring that your media plan is not only data-driven but also accurate and trustworthy. The iterative process of model calibration and validation allows for enhanced business performance and growth.
Get in touch with our Media Solutions team if you want to learn more about Media Mix Modelling and incrementality testing to support your business needs.